In order to achieve a sustainable nuclear fusion reactor, among other problems turbulence needs to be modeled and optimized. Enhanced crossfield transport is induced by turbulence which leads to degradation of confinement of a hot fusion plasma. Turbulence can be modelled with direct numerical simulation. However, due to the enormous span of time and spatial scales, involved in these calculations, are not fast enough for specific applications like modelling discharge timescales even with current supercomputers.
Reduced turbulence models have been created, such as QuaLiKiz, a quasilinear gyrokinetics code which is applicable for tokamak simulations. QuaLiKiz is still too slow for extensive optimization and control applications. Further speed up is possible by training of neural networks on reduced model input-output mapping. A critical part of neural network training is the population of the training data set, i.e. the input-output mapping. Under-population of the data set leads to enormous errors in the trained surrogate models. Over-population leads to wasted computational resources in creating the input-output mapping from the turbulence models.
General practice is to over-populate databases, which is still tractable when using models such as QuaLiKiz, but will be impossible with higher fidelity codes, such as GENE, due to limitations in computing power. Therefore this work focuses on the following research question: Can a robust methodology be developed for reducing training set size while maintaining sufficient data density for accurate surrogate model development?
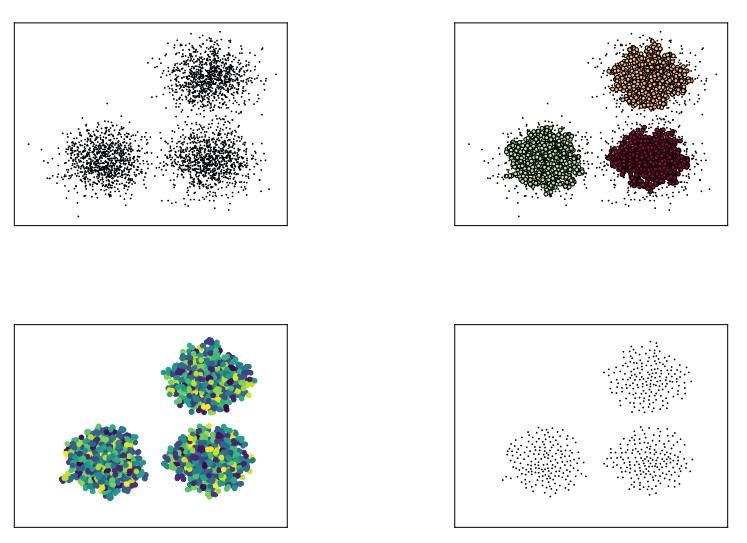